In the days before analytics existed, there was analogue closed-circuit television or CCTV, and it was up to the individuals monitoring these systems to identify as much as it is possible for a person to do. Then we had IP video, which saw surveillance systems evolve by enabling video transmission over network cable, and IP cameras that could be attached to networks simply and easily.
Here, a person could watch a camera feed that is focusing on a parking garage, for example. They could only tell that something suspicious is happening.
Not long after, developers realised that by using algorithms, digital video could be analysed automatically, and this became a popular feature of video management software, which now had the ability to pick up movement, identify how many people there were in a particular environment, and even set virtual tripwires.
All these analytics happened at the heart of the surveillance system, the network video recorder, or the server. This was very bandwidth heavy, as vast amounts of video needed to be transmitted before it could be analysed.
In its essence metadata is information about data
This is where the intelligent edge stepped in, and there was a shift towards more analytics taking place at the edge. This approach has the instant advantage of being able to analyse camera images at the source, without having to send bandwidth-heavy video across the network, instead, sending the results of the analysis to the virtual memory system as lightweight data.
The immediate benefits of edge-based analytics are considerable. With analytics taking place within the camera, at the edge of the network, only valuable data needs to be sent to the operator. Analysing video at the source, and as close as possible to where it is captured, means all images being examined are of the highest possible quality, with zero degradation caused by compressing images before transferring them.
Developers realised that by using algorithms, digital video could be analysed automatically
Moreover, edge devices, such as cameras and other sensors, now have more processing power, and have previously undreamed-of analytics capabilities. With analytics built in, cameras today can be taught to automatically detect sounds, activities, objects, and much more, putting real-time data in the security practitioner’s arsenal, and enabling them to make critical, and, importantly, accurate decisions. Not only does intelligent edge analytics turn video information into data, but it also creates metadata.
In its essence, metadata is information about data. Take a picture on your smartphone, for example. You will look at it and recognise a sunrise, clouds, or the ocean, but if you drill down into that data, you’ll be able to establish what time or where the picture was taken. Where the benefits come in, is that by harnessing both data and metadata, analysing floods of information becomes far easier and more effective.
All these analytics happened at the heart of the surveillance system
Edge technology has enabled users to fully harness the benefits of today’s analytics technology in a video and audio surveillance context. The modern chipsets we find today have given these devices a dramatic boost in processing power, enabling analytics to be loaded onto the devices themselves, which significantly lowers the amount of data that needs to be transmitted to cloud servers, and the reduction in bandwidth needs that has resulted from this has democratised analytics, putting it into the hands of those who could never have afforded it 10 years ago.
Concurrently, the rise of artificial intelligence (AI), machine learning, and deep learning have has made it easier to train new analytical models, which in turn makes them infinitely more accurate and reliable. In addition, these technologies are upgraded through software and firmware updates, dramatically adding to their relevance and lifespan. There is no longer a need to swap out a whole piece of hardware every time an upgrade is issued.
There was a shift towards more analytics taking place at the edge
We have now established that the edge devices we have today are smarter, quicker, and more intuitive and powerful, but what does that really mean for us? In terms of surveillance, it means increasingly less dependence on human intervention and a growing reliability on automated solutions. In turn, this translates into a significant reduction in false positives and an enhanced ability to filter out the noise that was inevitable with audio and video recordings.
Devices today can process exponentially more information, thereby generating the metadata we need to effectively categorise and cross-reference data across a business. The sheer volume of information also enables analytics to be more effectively trained for better accuracy, which again, reduces false alarms by allowing a camera to be able to tell the difference between a human transgressor and a neighbourhood cat on the prowl.
In addition, it is possible to train intelligent devices to recognise particular noises, such as glass breaking or gunfire, that are real signs a security incident is happening. Even voices that are raised, and aggressive behaviour can be used as triggers, informing security staff that a dangerous or unsafe incident might be taking place, which not only greatly reduces the time taken to respond to such incidents, but potentially prevents them from happening in the first place.
This approach has the instant advantage of being able to analyse camera images at the source
Urban parking management is another fantastic application. Network surveillance cameras, even ones already in use in a town or city, can be tweaked through specific analytics applications to not only alert traffic personnel to parking violations, but can show drivers where empty parking spaces are.
Detection zones that can be pre-defined could trigger alerts if a vehicle stops where it is not supposed to, such as in a loading zone, or stays somewhere for too long. Alerts could be sent to traffic enforcement to prevent congestion and fine violators.
Additional benefits could be realised through the connection of data and systems. One example would be combining payment apps and license plate recognition, enabling parking management systems to settle parking fees automatically. This would be a time saver too, allowing drivers to leave parking garages without having to queue to pay at the machines, and save them annoyance when the machines are out of order.
Moreover, by analysing real-time and historical data, it is possible to predict peak times, so parking lot owners can open extra spaces, or let drivers know in advance when it is likely to be full. By adding AI to the analysis, parking management solutions could even predict where drivers have the best chance of finding an open spot when they arrive.
Analysing video at the source means all images being examined are of the highest possible quality, with zero degradation caused by compressing images.
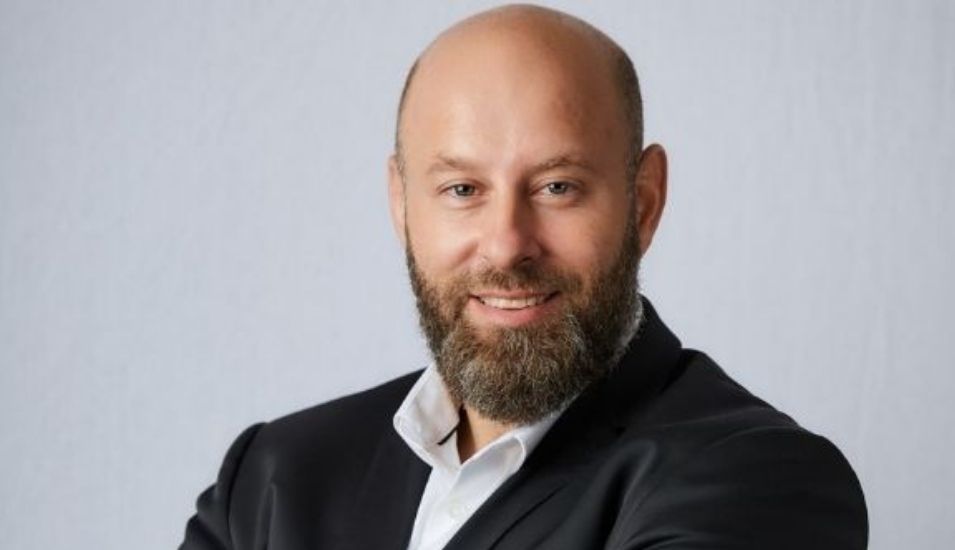