Technologists have now found innovative ways to overcome challenges to usher in what is being called the exascale era of computing. An exascale system is one that can perform a quintillion floating-point operations per second FLOPS. That’s a billion billion or 1,000,000,000,000,000,000 – which means exascale machines can solve calculations five times faster than today’s top supercomputers, and also run more complex, higher precision models.
To reach these new performance highs, engineers are taking a heterogeneous approach, consisting of integrated CPUs and GPUs and iterative optimisation of both hardware and software in order to reach new levels of performance and efficiency at a lower cost per FLOPS.
An exascale system is one that can perform a quintillion floating-point operations per second
Nowhere is this better demonstrated than with the Frontier supercomputer being developed at the Oak Ridge Leadership Computing Facility in the United States, which is set to make history as the world’s first operational exascale supercomputer when it is switched on later this year.
The machine, which will accelerate innovation in science and technology and help the US maintain leadership in high-performance computing and AI, is powered by 3rd-gen EPYC CPUs and Radeon Instinct GPUs and will deliver more than 1.5 exaflops of peak processing power. There is an even more powerful Exascale-class system, called El Capitan, anticipated to be built at Lawrence Livermore National Labs in 2023 in the United States.
Japan was first to market with its own FUGAKU 1.42 exaflops peak performance supercomputer and China is reportedly operating a less publicised Sunway Oceanlite 1.32 exaflops peak performance system.
That is a billion billion, which means exascale machines can solve calculations five times faster than today’s top supercomputers
Europe is taking a more collaborative approach with the government-funded European High-Performance Computing Joint Undertaking, which was initiated and pursued by the Partnership for Advanced Computing in Europe. The initiative pools resources to fund world-class integrated European high-performance computing and data infrastructure and support an innovative supercomputing ecosystem.
The continent’s supercomputing efforts is also bolstered by Horizon Europe, a seven-year European Union scientific research framework that is investing nearly €80 billion to fuel discoveries and world-firsts, including the development of EU-based exascale machines.
Take the Hawk supercomputer, currently the 24th system in the Top 500 list of world’s fastest supercomputers, installed at the University of Stuttgart HLRS. This machine – an HPE Apollo 9000 system with 5,632 nodes spread across its 44 cabinets, each node carrying EPYC CPUs – delivers around 26 peak petaflops of performance. HLRS is enabling customers in the automotive segment to run structural analysis and fluid dynamic simulations.
There’s also Lumi, a pre-exascale machine located at the IT Centre for Science CSC in Kajaani, Finland that demonstrates the power of this next era of supercomputing. Lumi, which utilises similar technology as Frontier with its custom EPYC Trento CPU and four Instinct MI250X GPU accelerators per node, will be capable of executing more than 375 petaflops or more than 375 million billion calculations per second, with a theoretical peak performance of more than 550 petaflops per second.
What makes exascale machines interesting is memory coherency
What makes pre and exascale machines particularly interesting is a memory coherency. This technology, not yet available to the general market, means there is a single copy of data accessed by both the CPU and GPUs, without the need to keep separate copies for each. This, in turn, reduces overhead programming, improves performance, and frees up system resources, helping bleeding edge systems like Lumi to run more efficiently.
Lumi also boasts innovative free cooling technology, which enables waste heat to be utilised in the district heating network of Kajaani, further reducing costs, and CO2 footprint. This technology is anticipated to reduce the entire city’s annual carbon footprint by 13,500 tons – an amount that equals the output from 4,000 passenger cars.
Thanks to this massive computational capacity, the machine – which already ranks amongst the world’s top supercomputers – is enabling European researchers to solve problems across different areas, from weather and cybersecurity through to drug discovery and personalised medicine. It is making breakthroughs in the area of climate change too; Lumi enables climate scientists to run high-resolution climate models, which can provide better insights for climate impact studies.
Memory coherency means there is a single copy of data accessed by both the CPU and GPUs
The continent now wants hardware that exceeds the performance of the world’s fastest Fugaku supercomputer in Japan. It is an ambitious and complex project and will take time, which is why the current democratisation of high-performance computing is so important.
It would require continuous focus and investments from multiple European nations to develop home-grown hardware, tools and scalable software if Europe is serious about operating its own, exascale class systems.
Supercomputers
Supercomputers, also called computational or parallel clusters process complex simulations by splitting compute problems into smaller jobs, simultaneously running on multiple server nodes, interconnected by one fast network. The price-performance of such systems is constantly improving, helping to make high performance computing more affordable.
Nowadays a compute problem that would take weeks to process on a multi-million-euro system twenty years ago can be accomplished in just a few hours on a single server, equipped with compute GPUs.
The hardware architecture with multiple computational GPUs used today in high performance computing space has many similarities with AI and ML implementations, and the intersection of the technologies is also bringing more advanced AI solutions to the mainstream, with high performance computing potentially enabling training models on ever larger datasets to optimise compute cluster use.
From 2002 to 2009, supercomputing performance doubled almost every 12 months. However, this rate dropped to every 2.3 years from 2009 to 2019, which has been credited to several factors such as the slowdown in Moore’s Law and technical constraints such as Dennard scaling.
To reach new performance highs, engineers are taking a heterogeneous approach, consisting of integrated CPUs and GPUs and iterative optimisation.
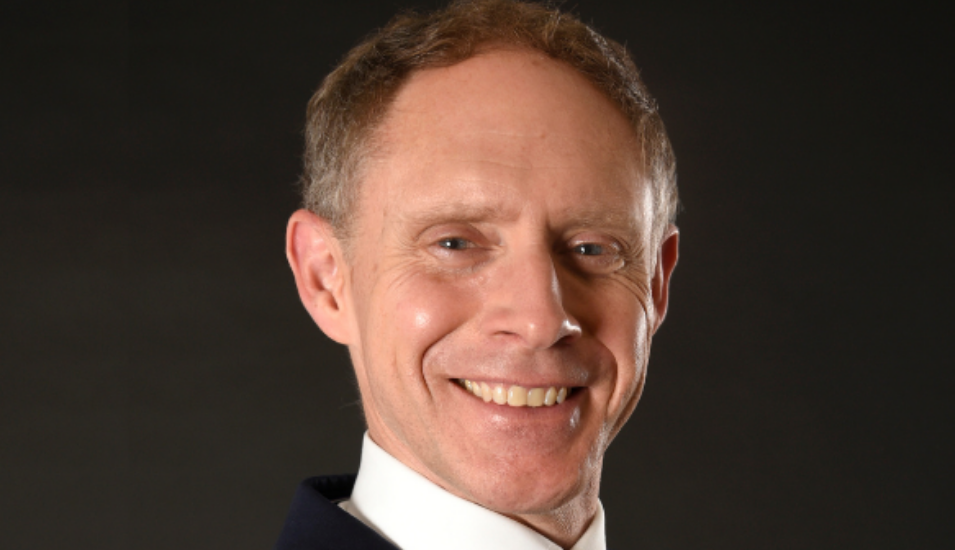